NextGen: HN — Digital Assessment: Adaptive assessment Proof of Concept - Case Study
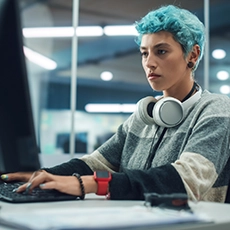
Qualification: HNC Computing
Proof of Concept
The Proof of Concept (PoC) aims to provide an opportunity for SQA to learn more about the possibilities and benefits of adaptive assessment and machine learning for NextGen: HN qualifications and inform next steps in SQA’s overall digital strategy. Computer Architecture (SCQF level 7) was selected for the adaptive assessment PoC, due to its assessment suitability and to enhance support during the independent learning stage. A formative assessment approach was adopted for this qualification, whereby the learner is assessed continuously throughout their learning journey instead of taking a single exam at the end.
Adaptive assessment is a personalised, dynamic learning journey based on the learner’s knowledge and understanding. This model moves away from linear assessment and sets out to tailor learning and assessment to individual needs. The process also provides more transparency regarding gaps in the learner’s knowledge for the assessor’s benefit.
Content development
We began the learning journey by looking at how to modify existing learning content and assessment to suit an adaptive approach as existing content may not have been suitable for an adaptive model. It was necessary to identify the core content that would enable the learner to achieve the unit, along with additional content that would enhance understanding of the concepts covered. The process also created the challenge of delivering the content in a logical and helpful sequence for the learner.
Glasgow Clyde College provided the PoC with original content which were adapted for incorporation into the Knowledge Space as stand-alone sections of learning. The content was supplemented with new content developed to ensure complete coverage of unit outcomes. The format and medium for all content required adjustment to satisfy the requirements of the adaptive model selected. A variety of question types were adopted for assessment, although the approach was limited to questions that could be machine marked. A set of assessment questions were selected for a pre-course diagnostic which informed the starting point in the Knowledge Space for each learner. The Knowledge Space is composed of a set of nodes, with each node being a single, standalone piece of learning content.
The proposed learning content and structure was reviewed by Walter Patterson, SQA Subject Implementation Manager of Computing. Walter identified the need for additional content, alongside additional assessment questions.
Digital solution
The digital solution identified for trialling this approach was Obrizum, a tool which uses artificial intelligence (AI) to automatically organise content into adaptive Knowledge Spaces. This tool takes individual learning resources and maps them into a Knowledge Space by using AI to analyse the content. Obrizum then delivers individualised content recommendations to learners and measures the impact on their knowledge and understanding through its question bank.
When answering an assessment question, the learner uses a confidence slider to indicate how confident they feel about their selected answer. This information is processed by Obrizum to provide a unique insight into the learner’s journey, which goes beyond traditional eLearning analytics. Obrizum processes insights including the average level of confidence in relation to a particular concept, along with the time taken to answer questions.
Learner trial
A key part of the PoC was to trial the approach with learners and college staff. Glasgow Clyde College helped us by trialling the solution with HNC Computing learners who were studying the Computer Architecture unit. The data from the use of the tool was used to explore the range of analytical capabilities.
The curriculum manager at the college shared his experience of using this tool.
‘It has potential to be a really good tool to go along with classroom teaching (it would suit some classes but not all). The students were really impressed at how it was adapting to how quickly they were picking up the material, they liked the dynamic nature of it. The confidence indicators were a good tool for both students and lecturers. From a teaching perspective, it’s good to see in an instance how the students are progressing and what material you would have to go back over.’
Following the trial, analytics including accuracy, confidence per concept, time taken to answer, user engagement, and content type were reviewed using a joint impact review.
The learner data was split into four key learner profiles: unaware learner, A* learner, beginner learner, and alpha learner.
The unaware learner may lack confidence in their knowledge, despite displaying a high level of accuracy in assessment. In contrast, the alpha learner consistently exhibits high confidence, regardless of the accuracy of their responses. This is valuable information that is not available in non-adaptive learning environments and gives the assessor further insight into areas which require additional work.
Findings and next steps
This PoC has identified opportunities and challenges related to this adaptive approach. Challenges included the creation of learning content suited to the structure of the Knowledge Space and ensuring logical sequencing. However, it makes content developers more aware of the linkages between learning items and the relationship with assessment, meaning it’s more focused on how learners progress in their understanding of key concepts. Overall, more consideration is required to establish the roles of humans and AI in the co-creation and adaptation of content.
As a result of the AI powered tool, some learners will see a different set of content from others, and also experience assessment differently. Due to the limitation of a small PoC, further insight into the AI concept and different tools would be needed to offer the required level of transparency of the process. We have identified benefits of an adaptive assessment approach but the suitability for non-linear learning requires significant consideration to inform future next steps.